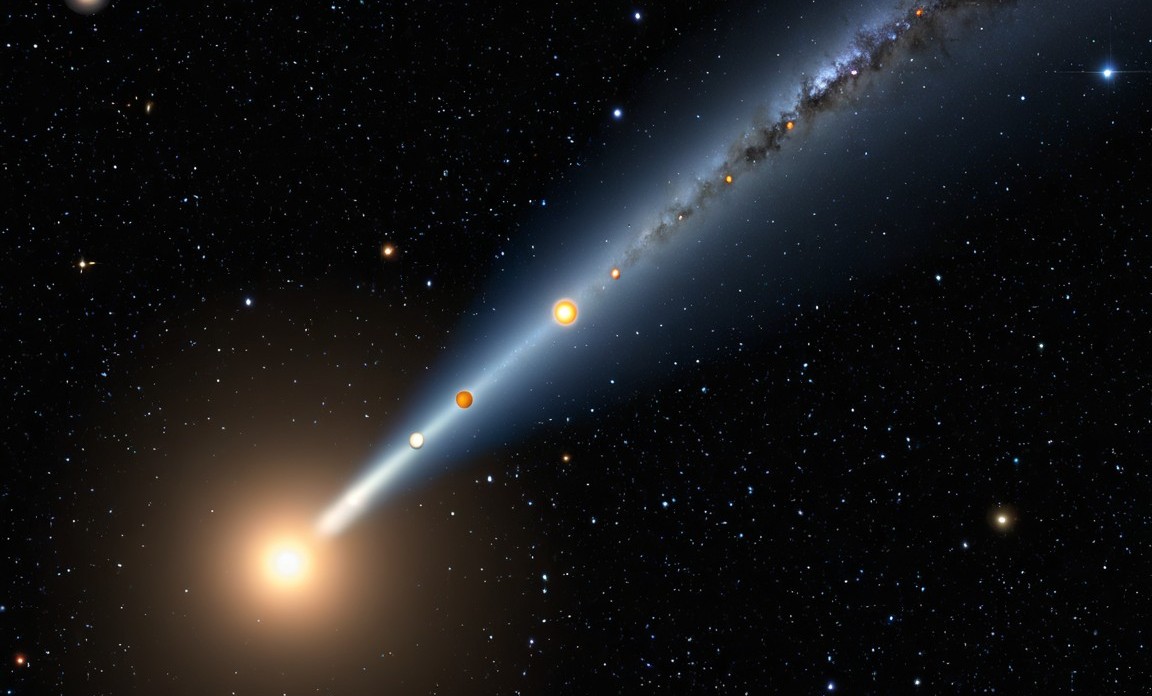
By using artificial intelligence (AI), scientists have improved the study of exoplanet atmospheres by the modeling of light interactions in exoplanet atmospheres to gain a better understanding of their chemical and physical characteristics.
Researchers can now more accurately model light scattering effects, especially from clouds, and gain a better understanding of exoplanet atmospheres by using physics-informed neural networks (PINNs).
This breakthrough, which is the product of collaboration between multiple top organizations worldwide, uses artificial intelligence (AI) to improve the analysis of spectral data from space, with a focus on recently acquired data from the James Webb Space Telescope.
Scientists from LMU, the ORIGINS Excellence Cluster, MPE, and ORIGINS Data Science Lab (ODSL) have made a major advancement in the analysis of exoplanet atmospheres through the use of physics-informed neural networks (PINNs). This has made it possible for them to more accurately model the intricate light scattering that occurs within these atmospheres than in the past, providing fresh perspectives on how clouds work and possibly significantly enhancing our comprehension of far-off worlds.
A tiny percentage of the starlight that exoplanets emit in front of their own star and an even smaller percentage of the planet’s atmosphere are blocked by exoplanets that are thousands of light years away from Earth. This causes changes in the light spectrum that represent the properties of the atmosphere (such as temperature, cloud cover, and chemical composition).
Scientists require models that can quickly compute millions of synthetic spectra in order to analyze the measured spectra. We can only provide information about the atmospheric composition of the observed exoplanetary bodies by comparing the calculated spectra with those of the exoplanets. Moreover, atmospheric models for the James Webb Space Telescope (JWST) must be equally intricate and detailed.
One major research concern is the scattering of light in exoplanets, especially the scattering of clouds. This phenomenon was not well captured by earlier models, which led to spectral analysis errors. On the other hand, neural networks with physics knowledge can easily solve complicated equations. Two models created by the researchers in the recent study were tested without light scattering and proved to be very accurate. The sky appears blue on Earth due to Rayleigh scattering, which was approximated in the second model.
A unique interdisciplinary collaboration between physicists from LMU Munich, the ORIGINS Excellence Cluster, MPE, and ORIGINS Data Science Lab (ODSL), which focuses on developing new AI-based methods in physics, enabled the development of these new discoveries.
The synergy not only advances exoplanet research but also creates new opportunities for AI-based techniques in physics, according to David Dahlbüdding, the study’s lead author at LMU. The partnership will enable the use of neural networks to simulate light scattering from clouds in a realistic manner, with an emphasis on expanding its interdisciplinary scope going forward.
The Royal Astronomical Society’s Monthly Notices, published in August 2024, contain a paper titled “Approximating Rayleigh scattering in exoplanetary atmospheres using physics-informed neural networks” by David Dahlbüdding, Karan Molaverdikhani, Barbara Ercolano and Tommaso Grassi. The DOI for the dataset is.10.1093/mnras/stae1872.